1. Initialize xo as any vector. Set ro = b - Axo and uo = zo = M-¹ro. 2. For k = 0, 1,...,n - 1: A. ak = u Auk B. Xк+1 = xk + akuk C. rk+1=rkak Auk D. if (k+1 < €): a. break E. Zk+1 = M-¹rk+1 +1²k+1 F. bk G. Uk+1 = Zk+1+bkuk 3. return *k+1.
1. Initialize xo as any vector. Set ro = b - Axo and uo = zo = M-¹ro. 2. For k = 0, 1,...,n - 1: A. ak = u Auk B. Xк+1 = xk + akuk C. rk+1=rkak Auk D. if (k+1 < €): a. break E. Zk+1 = M-¹rk+1 +1²k+1 F. bk G. Uk+1 = Zk+1+bkuk 3. return *k+1.
Database System Concepts
7th Edition
ISBN:9780078022159
Author:Abraham Silberschatz Professor, Henry F. Korth, S. Sudarshan
Publisher:Abraham Silberschatz Professor, Henry F. Korth, S. Sudarshan
Chapter1: Introduction
Section: Chapter Questions
Problem 1PE
Related questions
Question

Transcribed Image Text:Convergence of iterative methods like the Conjugate Gradient Method can be accelerated by the use of a technique called preconditioning. The convergence
rates of iterative methods often depend, directly or indirectly, on the condition number of the coefficient matrix A. The idea of preconditioning is to reduce the
effective condition number of the problem.
The preconditioned form of the n X n linear system Ax = b is
where M is an invertible n x n matrix called the preconditioner.
When A is a symmetric positive-definite n x n matrix, we will choose a symmetric positive-definite matrix M for use as a preconditioner. A particularly simple
choice is the Jacobi preconditioner M = D, where D is the diagonal of A. The Preconditioned Conjugate Gradient Method is now easy to describe:
Replace Ax = b with the preconditioned equation M-¹Ax = M-¹b, and replace the Euclidean inner product with (v, w) M.
To convert Algorithm 2 in Section 3.3 to the preconditioned version, let zk = M-¹b - M-¹ Axk = M¹. Then the algorithm is
1. Initialize x as any vector. Set ro = b - Axo and u。 = Zo = M-¹ro.
2. For k = 0,
1,..., n - 1:
rizk
A. ak =
u Auk
B. Jk+1 = k taklk
C. Tk+1=rk - ak Auk
D. if (k+1 < €):
a. break
E. Zk+1 = M-¹7k+1
F. bk =
THzk+1
rT Zk
G. Uk+1 = Zk+1 +bkuk
M¹Ax = M¹b,
3. return xk+1.

Transcribed Image Text:Now, consider the following problem.
Let A be the n x n matrix with n = 1000 and entries A(i, i) = i, A(i, i + 1) = A(i + 1, i) = 1/2, A(i, i + 2) = A(i + 2, i) = 1/2 for all i that fit within the
matrix.
(a) Take a look at the nonzero structure of the matrix using plt.spy(A).
(b) Let xe be the vector of n ones (exact solution). Set b = Axe, and apply the Conjugate Gradient Method, without preconditioner, and with the Jacobi
preconditioner. Compare errors (using 2-norm) of the two runs in a plot versus step number (using semilogy). (So you need to modify the conjugate gradient
codes to keep track of and return the solutions of all steps.) Use eps = 1e-10.
The two methods may converge in different number of steps. Which one do you see is faster?
Expert Solution

This question has been solved!
Explore an expertly crafted, step-by-step solution for a thorough understanding of key concepts.
Step by step
Solved in 3 steps with 3 images

Knowledge Booster
Learn more about
Need a deep-dive on the concept behind this application? Look no further. Learn more about this topic, computer-science and related others by exploring similar questions and additional content below.Recommended textbooks for you
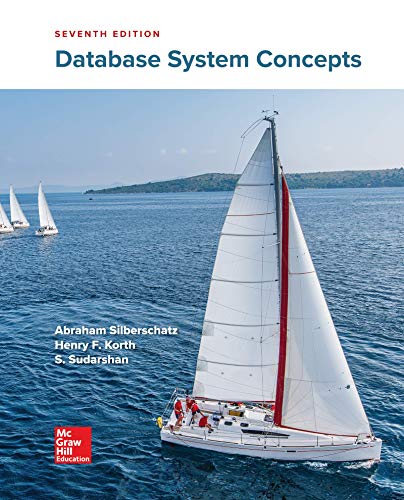
Database System Concepts
Computer Science
ISBN:
9780078022159
Author:
Abraham Silberschatz Professor, Henry F. Korth, S. Sudarshan
Publisher:
McGraw-Hill Education
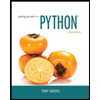
Starting Out with Python (4th Edition)
Computer Science
ISBN:
9780134444321
Author:
Tony Gaddis
Publisher:
PEARSON
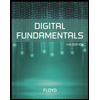
Digital Fundamentals (11th Edition)
Computer Science
ISBN:
9780132737968
Author:
Thomas L. Floyd
Publisher:
PEARSON
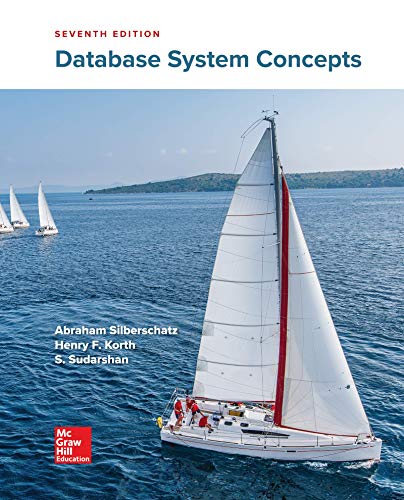
Database System Concepts
Computer Science
ISBN:
9780078022159
Author:
Abraham Silberschatz Professor, Henry F. Korth, S. Sudarshan
Publisher:
McGraw-Hill Education
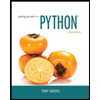
Starting Out with Python (4th Edition)
Computer Science
ISBN:
9780134444321
Author:
Tony Gaddis
Publisher:
PEARSON
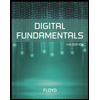
Digital Fundamentals (11th Edition)
Computer Science
ISBN:
9780132737968
Author:
Thomas L. Floyd
Publisher:
PEARSON
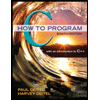
C How to Program (8th Edition)
Computer Science
ISBN:
9780133976892
Author:
Paul J. Deitel, Harvey Deitel
Publisher:
PEARSON
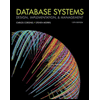
Database Systems: Design, Implementation, & Manag…
Computer Science
ISBN:
9781337627900
Author:
Carlos Coronel, Steven Morris
Publisher:
Cengage Learning
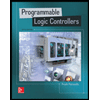
Programmable Logic Controllers
Computer Science
ISBN:
9780073373843
Author:
Frank D. Petruzella
Publisher:
McGraw-Hill Education